GPF Getting Started Guide
Prerequisites
This guide assumes that you are working on a recent Linux box.
Working version of anaconda or miniconda
The GPF system is distributed as an Anaconda package using the conda package manager.
If you do not have a working version of Anaconda or Miniconda, you must install one. We recommended using a Miniconda version.
Go to the Miniconda distribution page, download the Linux installer
wget -c https://repo.anaconda.com/miniconda/Miniconda3-latest-Linux-x86_64.sh
and install it in your local environment:
sh Miniconda3-latest-Linux-x86_64.sh
Note
At the end of the installation process, you will be asked if you wish
to allow the installer to initialize Miniconda3 by running conda init.
If you choose to, every terminal you open after that will have the base
Anaconda environment activated, and you’ll have access to the conda
commands used below.
Once Anaconda/Miniconda is installed, we would recommend installing mamba
instead of conda
. Mamba will speed up the installation of packages:
conda install -c conda-forge mamba
GPF Installation
The GPF system is developed in Python and supports Python 3.9 and up. The recommended way to set up the GPF development environment is to use Anaconda.
Install GPF
Create an empty Anaconda environment named gpf:
conda create -n gpf
To use this environment, you need to activate it using the following command:
conda activate gpf
Install the gpf_wdae conda package into the already activated gpf environment:
mamba install \
-c defaults \
-c conda-forge \
-c bioconda \
-c iossifovlab \
gpf_wdae
This command is going to install GPF and all of its dependencies.
Create an empty GPF instance
Create an empty directory named data-hg38-empty
:
mkdir data-hg38-empty
and inside it, create a file named gpf_instance.yaml
with the following
content:
reference_genome:
resource_id: "hg38/genomes/GRCh38-hg38"
gene_models:
resource_id: "hg38/gene_models/refSeq_v20200330"
This will create a GPF instance that:
The reference genome used by this GPF instance is
hg38/genomes/GRCh38-hg38
from default GRR;The gene models used by this GPF instance are
hg38/gene_models/refSeq_v20200330
from default GRR;If not specified otherwise, the GPF uses the default genomic resources repository located at https://www.iossifovlab.com/distribution/public/genomic-resources-repository/. Resources are used without caching.
Run the GPF development web server
By default, the GPF system looks for a file gpf_instance.yaml
in the
current directory (and its parent directories). If GPF finds such a file, it
uses it as a configuration for the GPF instance. Otherwise, it throws an
exception.
Now we can run the GPF development web server and browse our empty GPF instance:
wgpf run
and browse the GPF development server at http://localhost:8000
.
To stop the development GPF web server, you should press Ctrl-C
- the usual
keybinding for stopping long-running Linux commands in a terminal.
Warning
The development web server run by wgpf run
used in this guide
is meant for development purposes only
and is not suitable for serving the GPF system in production.
Import genotype variants
Data Storage
The GPF system uses genotype storages for storing genomic variants.
We are going to use in-memory genotype storage for this guide. It is easiest to set up and use, but it is unsuitable for large studies.
By default, each GPF instance has internal in-memory genotype storage.
Import Tools and Import Project
Importing genotype data into a GPF instance involves multiple steps. The tool used to import genotype data is named import_tools. This tool expects an import project file that describes the import.
This tool supports importing variants from three formats:
List of de novo variants
List of de novo CNV variants
Variant Call Format (VCF)
Example import of de novo variants: helloworld
Note
Input files for this example can be downloaded from
denovo-helloworld.tar.gz
.
Let us import a small list of de novo variants. We will need the list of
de novo variants helloworld.tsv
:
CHROM POS REF ALT person_ids
chr14 21403214 T C p1
chr14 21431459 G C p1
chr14 21391016 A AT p2
chr14 21403019 G A p2
chr14 21402010 G A p1
chr14 21393484 TCTTC T p2
and a pedigree file that describes the families helloworld.ped
:
familyId personId dadId momId sex status role phenotype
f1 m1 0 0 2 1 mom unaffected
f1 d1 0 0 1 1 dad unaffected
f1 p1 d1 m1 1 2 prb autism
f1 s1 d1 m1 2 2 sib unaffected
f2 m2 0 0 2 1 mom unaffected
f2 d2 0 0 1 1 dad unaffected
f2 p2 d2 m2 1 2 prb autism
Warning
Please note that the default separator for the list of de novo and pedigree
files is TAB
. If you copy these snippets and paste them into
corresponding files the separators between values most probably will
become spaces.
You need to ensure that separators between column values
are TAB
symbols.
The project configuration file for importing this study
denovo_helloworld.yaml
should look like:
id: denovo_helloworld
input:
pedigree:
file: helloworld.ped
denovo:
files:
- helloworld.tsv
person_id: person_ids
chrom: CHROM
pos: POS
ref: REF
alt: ALT
To import this project run the following command:
import_tools denovo_helloworld.yaml
When the import finishes you can run the GPF development server using:
wgpf run
and browse the content of the GPF development server at http://localhost:8000
Example import of VCF variants: vcf_helloworld
Note
Input files for this example can be downloaded from
vcf-helloworld.tar.gz
.
Let us have a small VCF file hellowrold.vcf
:
##fileformat=VCFv4.2
##FORMAT=<ID=GT,Number=1,Type=String,Description="Genotype">
##contig=<ID=chr14>
#CHROM POS ID REF ALT QUAL FILTER INFO FORMAT m1 d1 p1 s1 m2 d2 p2
chr14 21385738 . C T . . . GT 0/0 0/1 0/1 0/0 0/0 0/1 0/0
chr14 21385954 . A C . . . GT 0/0 0/0 0/0 0/0 0/1 0/0 0/1
chr14 21393173 . T C . . . GT 0/1 0/0 0/0 0/1 0/0 0/0 0/0
chr14 21393702 . C T . . . GT 0/0 0/0 0/0 0/0 0/0 0/1 0/1
chr14 21393860 . G A . . . GT 0/0 0/1 0/1 0/1 0/0 0/0 0/0
chr14 21403023 . G A . . . GT 0/0 0/1 0/0 0/1 0/1 0/0 0/0
chr14 21405222 . T C . . . GT 0/0 0/0 0/0 0/0 0/0 0/1 0/0
chr14 21409888 . T C . . . GT 0/1 0/0 0/1 0/0 0/1 0/0 1/0
chr14 21429019 . C T . . . GT 0/0 0/1 0/1 0/0 0/0 0/1 0/1
chr14 21431306 . G A . . . GT 0/0 0/1 0/1 0/1 0/0 0/0 0/0
chr14 21431623 . A C . . . GT 0/0 0/0 0/0 0/0 0/1 1/1 1/1
chr14 21393540 . GGAA G . . . GT 0/1 0/1 1/1 0/0 0/0 0/0 0/0
and a pedigree file helloworld.ped
(the same pedigree file used in
`Example import of de novo variants: ``helloworld```_):
familyId personId dadId momId sex status role phenotype
f1 m1 0 0 2 1 mom unaffected
f1 d1 0 0 1 1 dad unaffected
f1 p1 d1 m1 1 2 prb autism
f1 s1 d1 m1 2 2 sib unaffected
f2 m2 0 0 2 1 mom unaffected
f2 d2 0 0 1 1 dad unaffected
f2 p2 d2 m2 1 2 prb autism
Warning
Please note that the default separator for the VCF and pedigree
files is TAB
. If you copy these snippets and paste them into
corresponding files the separators between values most probably will
become spaces.
You need to ensure that separators between column values
are TAB
symbols for import to work.
The project configuration file for importing this VCF study
vcf_helloworld.yaml
should look like:
id: vcf_helloworld
input:
pedigree:
file: helloworld.ped
vcf:
files:
- helloworld.vcf
To import this project run the following command:
import_tools vcf_helloworld.yaml
When the import finishes you can run the GPF development server using:
wgpf run
and browse the content of the GPF development server at http://localhost:8000
Example of a dataset (group of genotype studies)
The already imported studies denovo_helloworld
and vcf_helloworld
have genomic variants for the same group of individuals helloworld.ped
.
We can create a dataset (group of genotype studies) that include both studies.
To this end create a directory datasets/helloworld
inside the GPF instance
directory data-hg38-empty
:
cd data-hg38-empty
mkdir -p datasets/helloworld
and place the following configuration file hellowrold.yaml
inside that
directory:
id: helloworld
name: Hello World Dataset
studies:
- denovo_helloworld
- vcf_helloworld
Example import of de novo variants from Rates of contributory de novo mutation in high and low-risk autism families
Let us import de novo variants from the Yoon, S., Munoz, A., Yamrom, B. et al. Rates of contributory de novo mutation in high and low-risk autism families. Commun Biol 4, 1026 (2021)..
We will focus on de novo variants from the SSC collection published in the aforementioned paper. To import these variants into the GPF system we need a list of de novo variants and a pedigree file describing the families. The list of de novo variants is available from Supplementary Data 2. The pedigree file for this study is not available. Instead, we have a list of children available from Supplementary Data 1.
Let us first export these Excel spreadsheets into CSV files. Let us say that the
list of de novo variants from the SSC collection is saved into a file named
SupplementaryData2_SSC.tsv
and the list of children is saved into a TSV file
named SupplementaryData1_Children.tsv
.
Note
Input files for this example can be downloaded from
denovo-in-high-and-low-risk-papter.tar.gz
.
Preprocess the families data
To import the data into GPF we need a pedigree file describing the structure
of the families. The SupplementaryData1_Children.tsv
contains only the list
of children. There is no information about their parents. Fortunately for the
SSC collection it is not difficult to build the full families’ structures from
the information we have. For the SSC collection if you have a family with ID
<fam_id>
, then the identifiers of the individuals in the family are going to
be formed as follows:
mother -
<fam_id>.mo
;father -
<fam_id>.fa
;proband -
<fam_id>.p1
;first sibling -
<fam_id>.s1
;second sibling -
<fam_id>.s2
.
Another important restriction for SSC is that the only affected person in the
family is the proband. The affected status of the mother, father and
siblings are unaffected
.
Using all these conventions we can write a simple python script
build_ssc_pedigree.py
to convert
SupplementaryData1_Children.tsv
into a pedigree file ssc_denovo.ped
:
"""Converts SupplementaryData1_Children.tsv into a pedigree file."""
import pandas as pd
children = pd.read_csv("SupplementaryData1_Children.tsv", sep="\t")
ssc = children[children.collection == "SSC"]
# list of all individuals in SSC
persons = []
# each person is represented by a tuple:
# (familyId, personId, dadId, momId, status, sex)
for fam_id, members in ssc.groupby("familyId"):
persons.append((fam_id, f"{fam_id}.mo", "0", "0", "unaffected", "F"))
persons.append((fam_id, f"{fam_id}.fa", "0", "0", "unaffected", "F"))
for child in members.to_dict(orient="records"):
persons.append((
fam_id, child["personId"], f"{fam_id}.fa", f"{fam_id}.mo",
child["affected status"], child["sex"]))
with open("ssc_denovo.ped", "wt", encoding="utf8") as output:
output.write(
"\t".join(("familyId", "personId", "dadId", "momId", "status", "sex")))
output.write("\n")
for person in persons:
output.write("\t".join(person))
output.write("\n")
If we run this script it will read SupplementaryData1_Children.tsv
and
produce the appropriate pedigree file ssc_denovo.ped
.
Preprocess the variants data
The SupplementaryData2_SSC.tsv
file contains 255231 variants. To import so
many variants in in-memory genotype storage is not appropriate. For this
example we are going to use a subset of 10000 variants:
head -n 10001 SupplementaryData2_SSC.tsv > ssc_denovo.tsv
Data import of ssc_denovo
Now we have a pedigree file ssc_denovo.ped
and a list of de novo
variants ssc_denovo.tsv
. Let us prepare an import project configuration
file ssc_denovo.yaml
:
id: ssc_denovo
input:
pedigree:
file: ssc_denovo.ped
denovo:
files:
- ssc_denovo.tsv
person_id: personIds
variant: variant
location: location
To import the study we should run:
import_tools ssc_denovo.yaml
and when the import finishes we can run the development GPF server:
wgpf run
In the list of studies, we should have a new study ssc_denovo
.
Getting started with Dataset Statistics
To generate family and de novo variant reports, you can use
the generate_common_report.py
tool. It supports the option --show-studies
to list all studies and datasets configured in the GPF instance:
generate_common_report.py --show-studies
To generate the reports for a given study or dataset, you can use the
--studies
option.
By default the dataset statistics are disabled. If we try to run
generate_common_report.py --studies helloworld
it will not generate the dataset statistics. Instead, it will print
a message that the reports are disabled to study helloworld
:
WARNING:generate_common_reports:skipping study helloworld
To enable the dataset statistics for the helloworld
dataset we need to
modify the configuration and add
a new section that enables dataset statistics:
id: helloworld
name: Hello World Dataset
studies:
- denovo_helloworld
- vcf_helloworld
common_report:
enabled: True
Let us now re-run the generate_common_report.py
command:
generate_common_report.py --studies helloworld
If we now start the GPF development server:
wgpf run
and browse the helloworld
dataset we will see the Dataset Statistics
section available.
Getting started with de novo gene sets
To generate de novo gene sets, you can use the
generate_denovo_gene_sets.py
tool. Similar to reports_tool above,
you can use the --show-studies
and --studies
option.
By default the de novo gene sets are disabled. If you want to enable them for a specific study or dataset you need to update the configuration and add a section that enable the de novo gene sets:
denovo_gene_sets:
enabled: true
For example the configuration of helloworld
dataset should become similar to:
id: helloworld
name: Hello World Dataset
studies:
- denovo_helloworld
- vcf_helloworld
common_report:
enabled: True
denovo_gene_sets:
enabled: true
Then we can generate the de novo gene sets for helloworld
dataset by
running:
generate_denovo_gene_sets.py --studies helloworld
Getting Started with Annotation
The import of genotype data into a GPF instance always runs effect annotation. It is easy to extend the annotation of genotype data during the import.
To define the annotation used during the import into a GPF instance we have to add a configuration file that defines the pipeline of annotators. After that, we need to configure the GPF instance to use this annotation pipeline.
There is a public Genomic Resources Repository (GRR) with a collection of public genomic resources available for use with GPF system.
Example: Annotation with GnomAD 3.0
To annotate the genotype variants with GnomAD allele frequencies we should
find the GnomAD genomic resource in our public GRR. We will choose to use
hg38/variant_frequencies/gnomAD_v3/genomes
resource. If we navigate
to the resource page we will see that this is an allele_score
resource.
So to use it in the annotation we should use the allele_score
annotator.
The minimal configuration of annotation with this GnomAD resource is the following:
- allele_score: hg38/variant_frequencies/gnomAD_v3/genomes
Store this annotation configuration in a file named annotation.yaml
and
configure the GPF instance to use this annotation configuration:
reference_genome:
resource_id: "hg38/genomes/GRCh38-hg38"
gene_models:
resource_id: "hg38/gene_models/refSeq_v20200330"
annotation:
conf_file: annotation.yaml
Now we can re-run the import for our helloworld
examples:
Go to the
denovo-helloworld
project directory and re-run the import:import_tools -f denovo_helloworld.yaml
Go to the
vcf-helloworld
project directory and re-run the import:import_tools -f vcf_helloworld.yaml
Once the re-import finishes, the variants in our Hello World Dataset
have
additional attributes that come from the annotation with GnomAD v3.0
. By
default annotation adds the following three attributes:
genome_gnomad_v3_af_percent
- allele frequencies as a percent;genome_gnomad_v3_ac
- allele count;genome_gnomad_v3_an
- number of sequenced alleles.
If we run the GPF development server and browse our Hello World Dataset
there are almost no difference. The only difference is that now in the
genotype browse the genomic scores section is not empty and we can query
our variants using the genome_gnomad_v3_af_percent
genomic score.
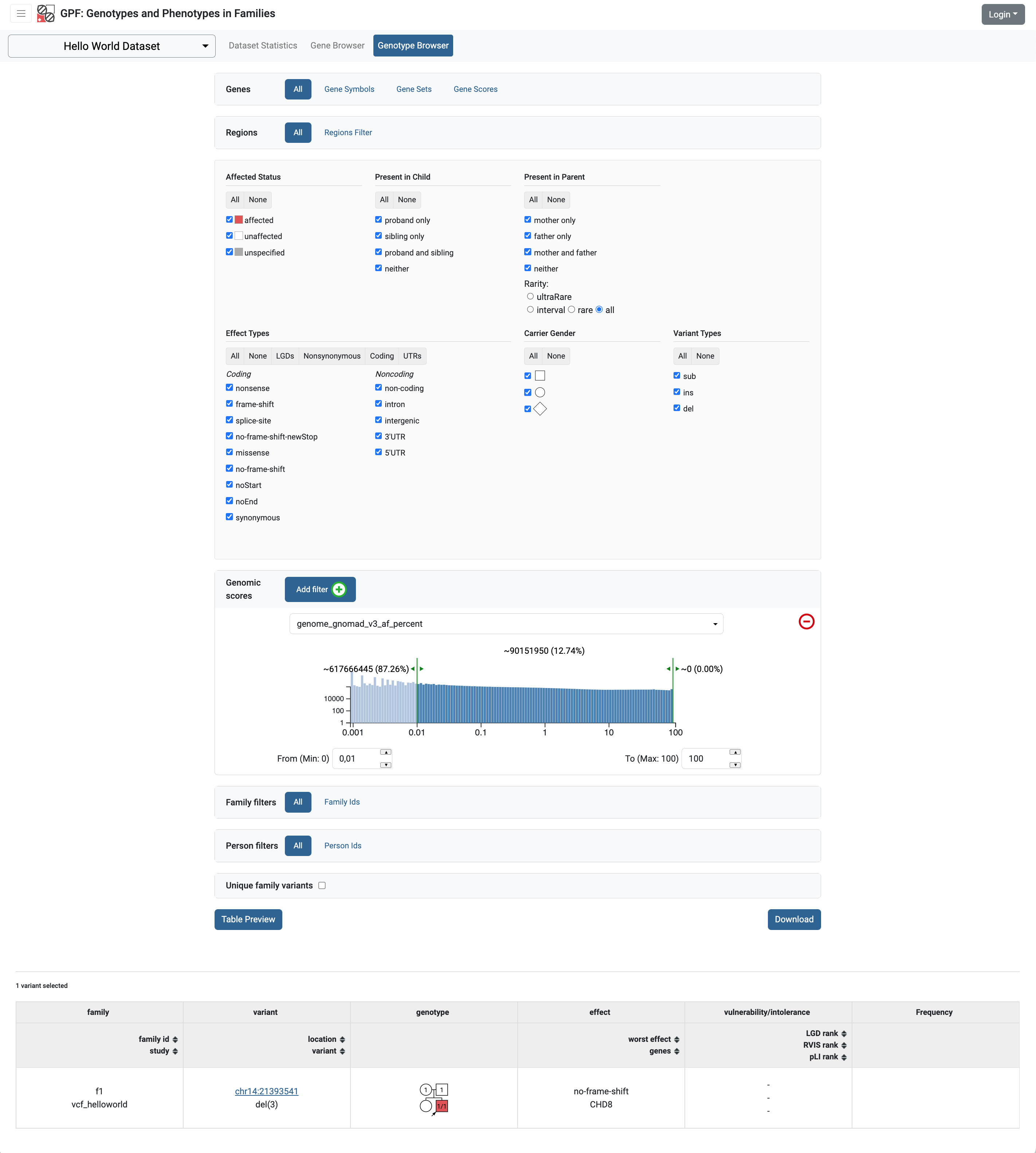
To make the new annotation attributes available in the variants preview table and in the variants download we need to change the study configuration. Check the Getting Started with Preview and Download Columns section for additional information.
Getting Started with Preview and Download Columns
When importing data into a GPF instance we can run an annotation pipeline that adds additional attributes to each variant. To make these attributes available in the variants preview table and in the variants download file we need to change the configuration of the corresponding study or dataset.
For each study dataset, you can specify which columns are shown in the variants’ table preview, as well as those which will be downloaded.
Example: Redefine the Frequency column in the preview table of Hello World Dataset`
As an example, we are going to redefine the Frequency column for helloworld
dataset to include attributes from annotation with GnomAD v3 genomic score.
Navigate to the helloworld
dataset folder:
cd datasets/helloworld
and edit the helloworld.yaml
file. Add the following section to the end:
genotype_browser:
columns:
genotype:
genome_gnomad_v3_af_percent:
name: gnomAD v3 AF
source: genome_gnomad_v3_af_percent
format: "%%.3f"
genome_gnomad_v3_ac:
name: gnomAD v3 AC
source: genome_gnomad_v3_ac
format: "%%d"
genome_gnomad_v3_an:
name: gnomAD v3 AN
source: genome_gnomad_v3_an
format: "%%d"
column_groups:
freq:
name: "Frequency"
columns:
- genome_gnomad_v3_af_percent
- genome_gnomad_v3_ac
- genome_gnomad_v3_an
This overwrites the definition of the default preview column Frequency to include the gnomAD v3 frequencies. If we now browse the Hello World Dataset and run variants preview in the genotype browser we will start seeing the GnomAD attributes:
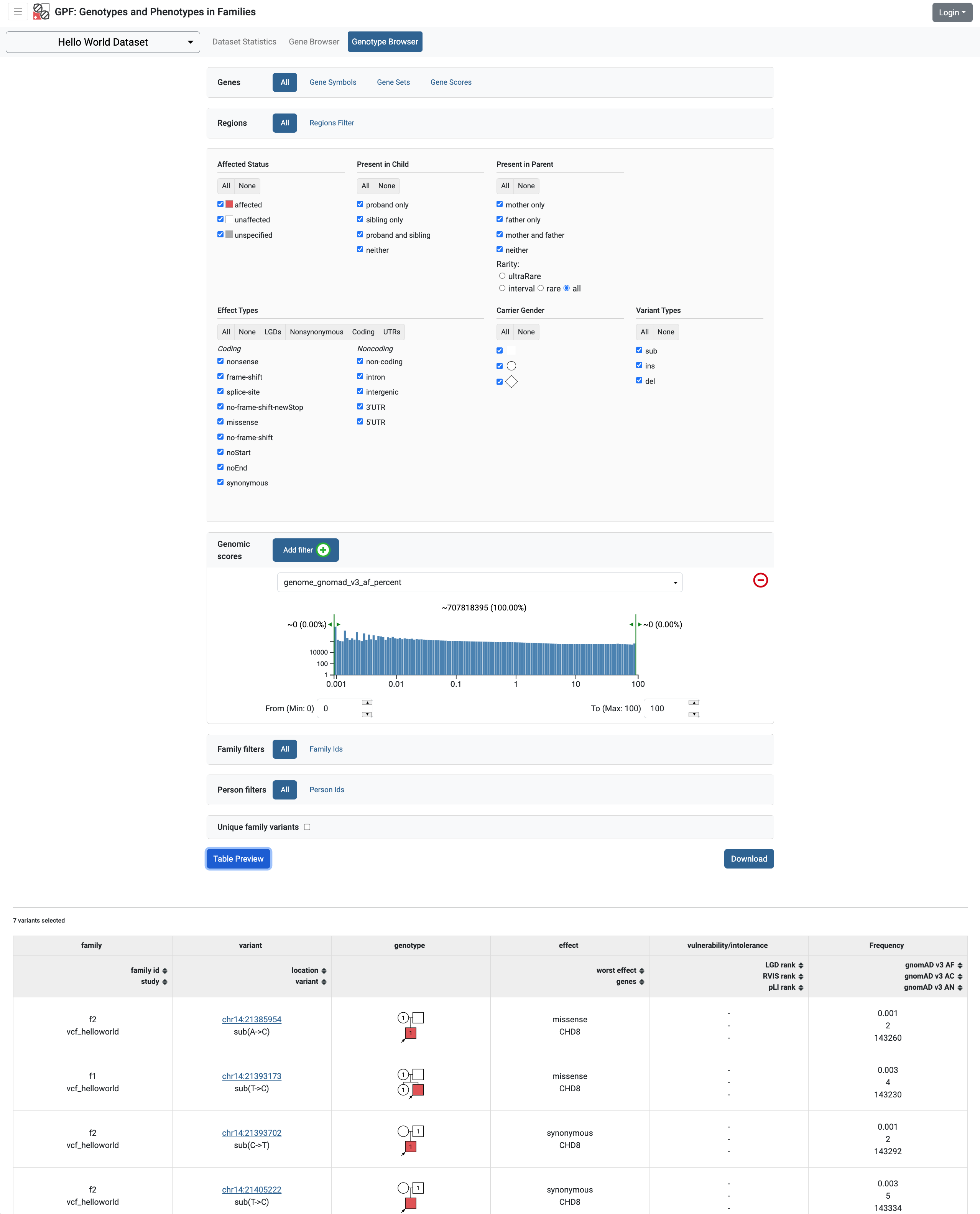
Example: Add GnomAD v3 columns to the variants download
As an example let us add GnomAD v3 columns to the variants downloads.
By default, each genotype study or dataset has a list of predefined columns used
when downloading variants. The users can replace the default list of download
columns by defining the download_columns
list or they can extend the predefined
list of download columns by defining the download_columns_ext
list of columns.
In the example below we are going to use download_columns_ext
to add
GnomAD v3 columns to the properties of downloaded variants:
genotype_browser:
columns:
genotype:
genome_gnomad_v3_af_percent:
name: gnomAD v3 AF
source: genome_gnomad_v3_af_percent
format: "%%.3f"
genome_gnomad_v3_ac:
name: gnomAD v3 AC
source: genome_gnomad_v3_ac
format: "%%d"
genome_gnomad_v3_an:
name: gnomAD v3 AN
source: genome_gnomad_v3_an
format: "%%d"
column_groups:
freq:
name: "Frequency"
columns:
- genome_gnomad_v3_af_percent
- genome_gnomad_v3_ac
- genome_gnomad_v3_an
download_columns_ext:
- genome_gnomad_v3_af_percent
- genome_gnomad_v3_ac
- genome_gnomad_v3_an
Getting Started with Gene Browser
The Gene Browser in the GPF system uses the allele frequency as a Y-coordinate when displaying the allele. By default, the allele frequency used is the frequency of the alleles in the imported data.

After annotation of the helloworld
data with GnomAD v3 we can use the GnomAD
allele frequency in the Gene Browser.
Example: configure the gene browser to use gnomAD frequency as the variant frequency
To configure the Hello World Dataset to use GnomAD v3 allele frequency
we need to add a new section
gene_browser
in the configuration file of the datasets
datasets/helloworld/helloworld.yaml
as follows:
id: helloworld
name: Hello World Dataset
...
gene_browser:
frequency_column: genome_gnomad_v3_af_percent
If we restart the GPF development server and navigate to Hello World Dataset
Gene Browser, the Y-axes will use the GnomAD allele frequency instead of the
study allele frequency.

Todo
WIP
Getting Started with Enrichment Tool
For studies that include de Novo variants, you can enable the enrichment_tool_ui. As an example, let us enable it for the already imported iossifov_2014 study.
Go to the directory where the configuration file of the iossifov_2014 study is located:
cd gpf_test/studies/iossifov_2014
Edit the study configuration file iossifov_2014.conf
and add the following section in the end of the file:
[enrichment]
enabled = true
Restart the GPF web server:
wdaemanage.py runserver 0.0.0.0:8000
Now when you navigate to the iossifov_2014 study in the browser, the Enrichment Tool tab will be available.
Getting Started with Phenotype Data
Simple Pheno Import Tool
The GPF simple pheno import tool prepares phenotype data to be used by the GPF system.
As an example, we are going to show how to import simulated phenotype data into our GPF instance.
Download the archive and extract it outside of the GPF instance data directory:
wget -c https://iossifovlab.com/distribution/public/pheno/phenotype-comp-data-latest.tar.gz
tar zxvf phenotype-comp-data-latest.tar.gz
Navigate to the newly created comp-data
directory:
cd comp-data
Inside you can find the following files:
comp_pheno.ped
- the pedigree file for all families included into the databaseinstruments
- directory, containing all instrumentsinstruments/i1.csv
- all measurements for instrumenti1
comp_pheno_data_dictionary.tsv
- descriptions for all measurementscomp_pheno_regressions.conf
- regression configuration file
To import the phenotype data, you can use the simple_pheno_import.py
tool. It will import
the phenotype database directly to the DAE data directory specified in your environment:
simple_pheno_import.py \
-p comp_pheno.ped \
-d comp_pheno_data_dictionary.tsv \
-i instruments/ \
-o comp_pheno \
--regression comp_pheno_regressions.conf
Options used in this command are as follows:
-p
specifies the pedigree file-d
specifies the name of the data dictionary file for the phenotype database-i
specifies the directory where the instruments are located-o
specifies the name of the output phenotype database that will be used in the Phenotype Browser--regression
specifies the path to the pheno regression config, describing a list of measures to make regressions against
You can use the -h
option to see all options supported by the tool.
Configure Phenotype Database
Phenotype databases have a short configuration file which points
the system to their files, as well as specifying additional properties.
When importing a phenotype database through the
simple_pheno_import.py
tool, a configuration file is automatically
generated. You may inspect the gpf_test/pheno/comp_pheno/comp_pheno.conf
configuration file generated from the import tool:
[vars]
wd = "."
[phenotype_data]
name = "comp_pheno"
dbfile = "%(wd)s/comp_pheno.db"
browser_dbfile = "%(wd)s/browser/comp_pheno_browser.db"
browser_images_dir = "%(wd)s/browser/images"
browser_images_url = "/static/comp_pheno/browser/images/"
[regression.age]
instrument_name = "i1"
measure_name = "age"
display_name = "Age"
jitter = 0.1
[regression.iq]
instrument_name = "i1"
measure_name = "iq"
display_name = "Non verbal IQ"
jitter = 0.1
Configure Phenotype Browser
To demonstrate how a study is configured with a phenotype database, we will
be working with the already imported comp_all
study.
The phenotype databases can be attached to one or more studies and/or datasets.
If you want to attach the comp_pheno
phenotype
database to the comp_all
study, you need to specify it in the study’s
configuration file, which can be found at gpf_test/studies/comp_all/comp_all.conf
.
Add the following line at the beginning of the file, outside of any section:
phenotype_data = "comp_pheno"
To enable the phenotype_browser_ui, add this line:
phenotype_browser = true
After this, the beginning of the configuration file should look like this:
id = "comp_all"
conf_dir = "."
has_denovo = true
phenotype_browser = true
phenotype_data = "comp_pheno"
When you restart the server, you should be able to see the ‘Phenotype Browser’ tab in the comp_all study.
Configure Family Filters in Genotype Browser
A study or a dataset can have phenotype filters configured for its genotype_browser_ui when it has a phenotype database attached to it. The configuration looks like this:
[genotype_browser]
enabled = true
family_filters.sample_continuous_filter.name = "Sample Filter Name"
family_filters.sample_continuous_filter.from = "phenodb"
family_filters.sample_continuous_filter.source_type = "continuous"
family_filters.sample_continuous_filter.filter_type = "multi"
family_filters.sample_continuous_filter.role = "prb"
After adding the family filters configuration, restart the web server and navigate to the Genotype Browser. You should be able to see the Advanced option under the Family Filters - this is where the family filters can be applied.
Configure Phenotype Columns in Genotype Browser
Phenotype columns contain values from a phenotype database. These values are selected from the individual who has the variant displayed in the genotype_browser_ui’s table preview. They can be added when a phenotype database is attached to a study.
Let’s add a phenotype column. To do this, you need to define it in the study’s config, in the genotype browser section:
[genotype_browser]
(...)
selected_pheno_column_values = ["pheno"]
pheno.pheno.name = "Measures"
pheno.pheno.slots = [
{role = "prb", source = "i1.age", name = "Age"},
{role = "prb", source = "i1.iq", name = "Iq"}
]
For the phenotype columns to be in the Genotype Browser table preview or download file,
they have to be present in the preview_columns
or the download_columns
in the Genotype Browser
configuration. Add this in the genotype_browser section:
preview_columns = ["family", "variant", "genotype", "effect", "weights", "mpc_cadd", "freq", "pheno"]
Enabling the Phenotype Tool
To enable the phenotype_tool_ui for a study, you must edit
the study’s configuration file and set the appropriate property, as with
the phenotype_browser_ui. Open the configuration file comp_all.conf
and add the following line:
phenotype_tool = true
After editing, it should look like this:
id = "comp_all"
conf_dir = "."
has_denovo = true
phenotype_browser = true
phenotype_data = "comp_pheno"
phenotype_tool = true
Restart the GPF web server and select the comp_all
study.
You should see the phenotype_tool_ui tab. Once you have selected it, you
can select a phenotype measure of your choice. To get the tool to acknowledge
the variants in the comp_all
study, select the All option of the
Present in Parent field. Since the effect types of the variants in the comp
study are only Missense and Synonymous, you may wish to de-select the
LGDs option under the Effect Types field. There are is also the option to
normalize the results by one or two measures configured as regressors - age and
non-verbal IQ.
Click on the Report button to produce the results.
Using Apache Impala as storage
Starting Apache Impala
To start a local instance of Apache Impala you will need an installed Docker.
Note
If you are using Ubuntu, you can use the following instructions to install Docker.
We provide a Docker container with Apache Impala. To run it, you can use the script:
run_gpf_impala.sh
This script pulls out the container’s image from dockerhub and runs it under the name “gpf_impala”. When the container is ready, the script will print the following message:
...
===============================================
Local GPF Apache Impala container is READY...
===============================================
Note
In case you need to stop this container, you can use the command docker stop gpf_impala
.
For starting the container, use run_gpf_impala.sh
.
Note
Here is a list of some useful Docker commands:
docker ps
shows all running docker containers
docker logs -f gpf_impala
shows the log from the “gpf_impala” container
docker start gpf_impala
starts the “gpf_impala” container
docker stop gpf_impala
stops the “gpf_impala” container
docker rm gpf_impala
removes the “gpf_impala” container (only if stopped)
Note
The following ports are used by the “gpf_impala” container:
8020 - for accessing HDFS
9870 - for Web interface to HDFS Named Node
9864 - for Web interface to HDFS Data Node
21050 - for accessing Impala
25000 - for Web interface to Impala daemon
25010 - for Web interface to Impala state store
25020 - for Web interface to Impala catalog
Please make sure these ports are not in use on the host where you are going to start the “gpf_impala” container.
Configuring the Apache Impala storage
The available storages are configured in DAE.conf
.
This is an example section which configures an Apache Impala storage.
[storage.test_impala]
storage_type = "impala"
dir = "/tmp/test_impala/studies"
impala.hosts = ["localhost"]
impala.port = 21050
impala.db = "gpf_test_db"
hdfs.host = "localhost"
hdfs.port = 8020
hdfs.base_dir = "/user/test_impala/studies"
Importing studies into Impala
The simple study import tool has an optional argument to specify the storage
you wish to use. You can pass the ID of the Apache Impala storage configured
in DAE.conf
earlier.
--genotype-storage <genotype storage id>
Id of defined in DAE.conf genotype storage [default:
genotype_impala]
For example, to import the IossifovWE2014 study into the “test_impala” storage, the following command is used:
simple_study_import.py IossifovWE2014.ped \
--id iossifov_2014 \
--denovo-file IossifovWE2014.tsv \
--genotype-storage test_impala
Example Usage of GPF Python Interface
The simplest way to start using GPF’s Python API is to import the GPFInstance
class and instantiate it:
from dae.gpf_instance.gpf_instance import GPFInstance
gpf_instance = GPFInstance()
This gpf_instance
object groups together a number of objects, each dedicated
to managing different parts of the underlying data. It can be used to interact
with the system as a whole.
For example, to list all studies configured in the startup GPF instance, use:
gpf_instance.get_genotype_data_ids()
This will return a list with the ids of all configured studies:
['comp_vcf',
'comp_denovo',
'comp_all',
'iossifov_2014']
To get a specific study and query it, you can use:
st = gpf_instance.get_genotype_data('comp_denovo')
vs = list(st.query_variants())
Note
The query_variants method returns a Python iterator.
To get the basic information about variants found by the query_variants
method,
you can use:
for v in vs:
for aa in v.alt_alleles:
print(aa)
1:865664 G->A f1
1:865691 C->T f3
1:865664 G->A f3
1:865691 C->T f2
1:865691 C->T f1
The query_variants
interface allows you to specify what kind of variants
you are interested in. For example, if you only need “splice-site” variants, you
can use:
st = gpf_instance.get_genotype_data('iossifov_2014')
vs = st.query_variants(effect_types=['splice-site'])
vs = list(vs)
print(len(vs))
>> 87
Or, if you are interested in “splice-site” variants only in people with “prb” role, you can use:
vs = st.query_variants(effect_types=['splice-site'], roles='prb')
vs = list(vs)
len(vs)
>> 62